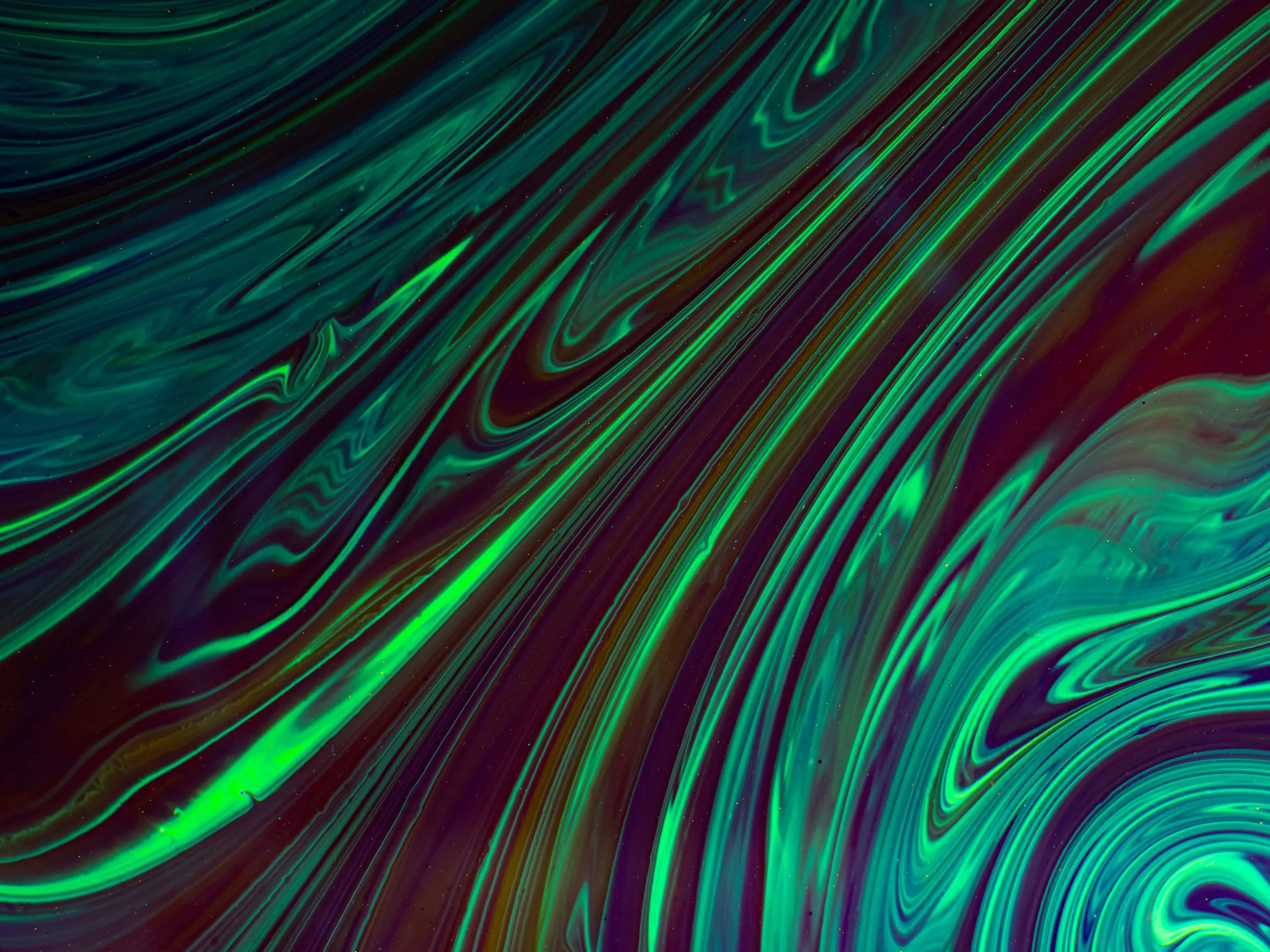
Zoltar
IVADO Labs, 2019-2020
Zoltar is an artificial intelligence (AI) price optimizer built by IVADO Labs for a Fortune 500 fuel retailer. It is a state-of-the-art bi-level demand forecaster and price optimizer leveraging cutting-edge machine learning (ML) and operations research (OR) techniques. The tool was built in 12 months by a cross-functional team composed of professors, data scientists, data engineers, and product managers. Zoltar has successfully undergone a three-month pilot before its global roll-out. It's estimated impact is >$100MM. Zoltar was developed in collaboration with professors from Université de Montréal and École Polytechnique de Montréal.
As an AI consultant, I was the integrator of our multi-disciplinary team. My role was to collaborate with the client to establish a precise list of functional requirements. Based on this list, I worked with the IVADO Labs development team to design and test the Zoltar scientific approach. During this iterative process, I was also in charge of communicating the methodology and results to the client.
During the project, the Zoltar team leveraged a user-centered approach. I focused my efforts on understanding the perspective of end-users and consumers to prioritize the development of specific features. As we modelled consumer behaviour, my input was critical for Zoltar feature engineering and explainability indicator design. Also, my ability to efficiently communicate abstract scientific concepts was an important enabler of our success. It facilitated client engagement and helped the team prioritize high-value features.
The Zoltar methodology
Zoltar uses a large number of variables to optimize fuel prices. It is designed to seamlessly push recommendations to existing pricing systems, thus limiting process changes.
Piloting Zoltar
During the proof of concept phase of the project, Zoltar underwent a three-month pilot during which fuel analysts used it to price multiple fuel types across five different business units of Europe and North America. The pilot was an opportunity to work with end-users and tune the model. This step was crucial to Zoltar's success; it allowed to ensure a granular alignment with context-specific consumer behaviour.